- Tags
- Article
- Creative and UX
- AI
A User-First Approach to AI (Harqen Case Study)
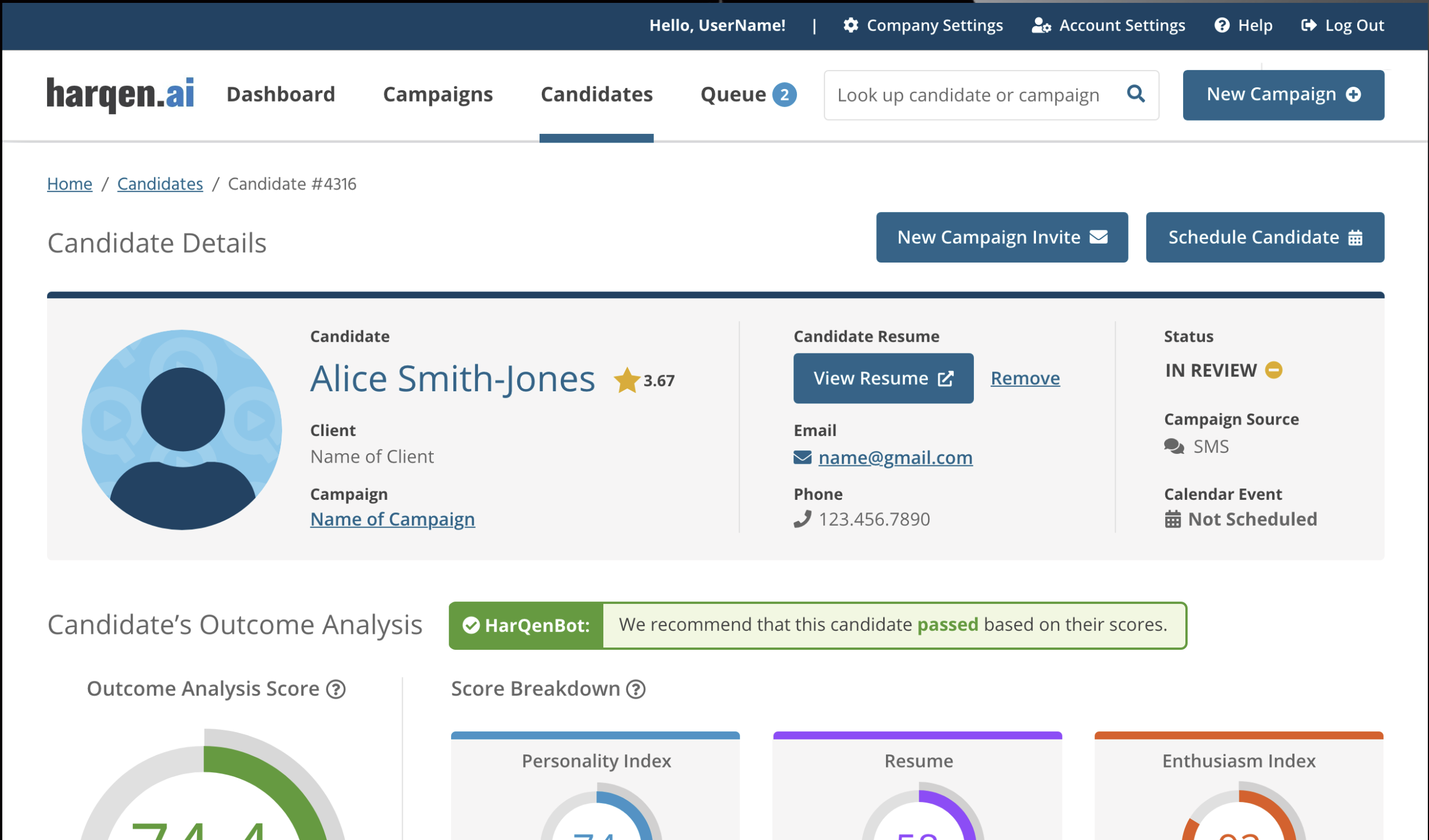
When considering any software solution, the first step is to identify who the users are and what problem they’re trying to solve. Artificial Intelligence is a powerful tool that, when correctly applied, can enhance a user’s experience and save them time and effort.
The Problem
In the case of Harqen, these users were recruiters and hiring managers handling a large volume of candidates. Recruiters have several candidates to manage at various stages of the interview process and need a scannable, intuitive UI that gives them the most important information at a glance. There was an additional opportunity to increase the efficiency of reviewing the candidate's interview responses and resumes, and overall determining if the candidate is the "right fit" for the job, through automation. By lowering the costs of the candidate review, while also speeding up the process of decision making, more can be accomplished in less time.
Exploring Solutions
Harqen wanted to improve the recruiters’ experience by analyzing data to determine the likelihood of success in a candidate filling a position and visually providing the results of that analysis on a user interface. In order to help automate candidate review, the solution would need to quantify a candidate and standardize the "fuzzy" subject data points such as personality. Harqen created a recruiting interview product that collected audio, video, and transcribed data from a candidate's interview and allowed for recruiters to track a candidate during the review process of the collected data.
For this solution, Harqen used a transcription service called Trint for the audio files because it provided an existing medical terminology dictionary that could recognize the medical terms used by candidates, which aligned with Harqen's main target market of healthcare interview candidates. Special models within Trint represented the varying dialects (which included industry and geo location specifications). GCP Mongo database was utilized as a JSON repository to store transcription information because it provided extensible storage allowing for interviews with varying number of questions and answer lengths
AI Analysis
Within the solution, candidate responses to interview questions are analyzed against previously Accepted or Declined candidates to determine likelihood for success on a number of metrics.
Personality
Personality traits were highly correlated with likeliness of acceptance by recruiters in many types of roles, ranging from flight attendants to ICU nurses. With a min of 500 words, the SAAS Receptivity could produce 80 different personality metadata scores ranging from persistence to curiosity. The product analyzes the text of the interview responses, not only focusing on what people say, but the context in which they say it to provide 9 discrete index and an overall composite score: Clout, Analytical thinking, Emotional tone, Openness, Conscientiousness, Extraversion, Agreeableness, Neuroticism, and Delayed Gratification (a custom personality metadata index created by Harqen representing a blend of traits including organization ability and future orientation).
Sentiment/Enthusiasm
Using the text of the audio interview, Harqen evaluated the text to identify positive vs negative words which are correlated to the successful vs unsuccessful hiring outcome of a candidate. The desired sentiment varies by industry – for example, the ideal sentiment for nurses may be “compassion” while the sentiment for security guards could be “calmness.” Using 5000+ words and their derivatives ranked from +5 as most enthusiastic to -5 for least, the Sentiment service provided a score for all text spoken by the candidate. On final analysis the Sentiment metadata score provided around 10% of the input to the final recommendation score.
Predictive Outcomes
The machine learning Data Robot provided an algorithm that could analyze text finding words and word clusters associated with successful/unsuccessful hiring outcomes. Gradient boosting is a machine learning technique used in regression and classification tasks, among others. It gives a prediction model in the form of an ensemble of weak prediction models which are typically decision trees. When a decision tree is the weak learning, the resulting algorithm is called gradient-boosted trees; it usually outperforms random forest. A gradient boosted trees model is built in a stage wise fashion, as in other boosting methods, but generalizes the other methods by allowing optimization of an arbitrary differentiable loss function.
Right/Wrong Answers
Harqen wanted to determine if the candidate answered the audio/video essay questions with a correct answer. The data robot identified words and word clusters that were associated with successful/unsuccessful hiring outcomes and provided a higher overall score for candidates that answered audio essay questions correctly.
Resume Analysis
Harqen wanted to analyze the legitimacy of the data of a candidate's resume to incorporate it into the overall outcome analysis by looking at the candidate's skills, experience, certifications, job description to resume description, and industries. For positions like ICU nurses, experience and certifications for specific procedures and equipment were requirements for hiring and were highly correlated to successful hiring outcomes. To process this data, SaaS Sovren parsed the resume into a JSON response.
Results
Harqen accumulated all metadata responses from the different analysis services into a GCP Mongo repo and built an overall interview model template combining all source and metadata inputs into a combined interview profile. The profile was submitted to Data Robot as a dataset to create the recommendation model.
Harqen achieved a 99.5% match between the Data Robot Recommendation model and the actual recruiter hiring outcomes from the results of 1.5 million interviews crossing a wide range of positions, including flight attendants, phlebotomists, ICU nurses, and security guards, based on actual historical data.
Creating the Product
Harqen partnered with nvisia to develop both a workflow service to create all of the interview metadata and modernize the legacy system to display the new AI data elements. We used GCP Publish/Subscribe for queueing and workflow software from Flowable to process the prepare/execute/post functions for each SaaS endpoint. Harqen and nvisia created over 200 REST endpoints and Angular front end services to display the new Outcome Analysis metadata to the users
Visualization for Comprehensive Data
Quality data visualization enables users to identify trends and patterns more quickly which saves users and businesses time and money. Before deciding on a visualization, first, understand how your users want to interact with the data. Are they needing to compare data points? Are they looking at parts of a whole? Focus on highlighting areas of importance to bring awareness, be mindful of drilling too deeply into the details to keep things clean and focused, and consider the accessibility of the visual display (colors, textures, labels, etc.).
ROI
Harqen rolled out the AI service in partnership with one of its largest customers for ICU travel nurse recruitment during the COVID epidemic and successfully introduced the service for a range of nursing and healthcare positions with this customer. Operational costs were $2.32/interview recommendation, one third of the original cost to review. Capital costs were spread over 3 years and added just $3/interview (or $30-50 per interview campaign). Based on a history of over 500K interviews, Harqen was able to create a model that accurately matched human recruiters with over 99% accuracy.
Starting Your AI Journey
If you need help guiding your future AI concepts, nvisia is here to help you on that journey. Through direct advisory services, workshops to help form your idea or assistance with implementation of an idea you have - we can help you! Find out more about our AI workshops or contact us to get started.